Factor analysis in research (Types, Assumptions, Characteristics, and Applications)
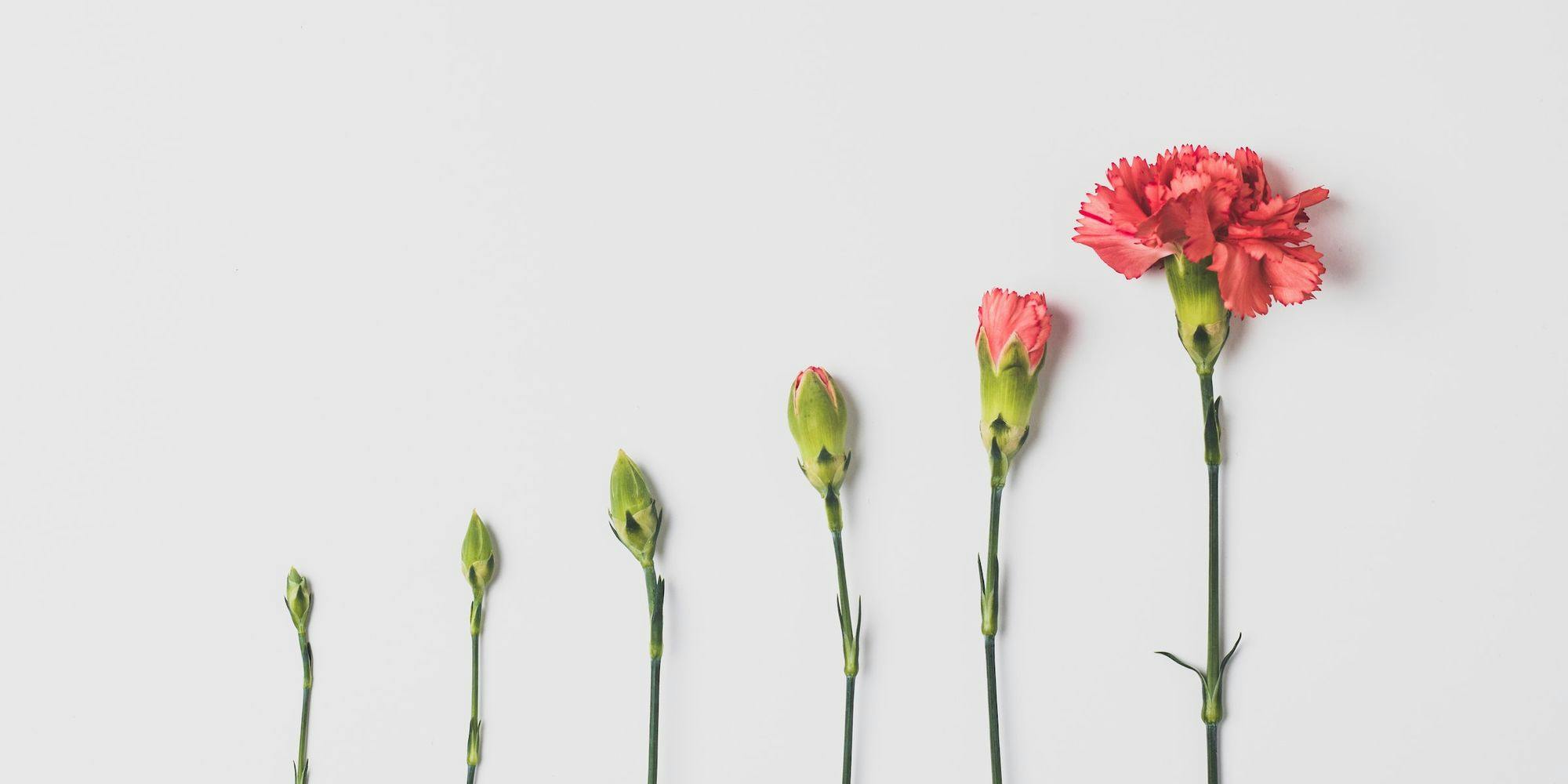
Factor analysis is a data reduction technique that identifies underlying dimensions, or factors, that explain the correlations among a set of variables. (Grove, Burns, & Gray, 2015, p. 218)
The basic idea of factor analysis is to identify a smaller number of latent variables or factors that can account for the covariation among the observed variables. These factors are not directly observed but are inferred from the observed variables based on their patterns of correlation. Factor analysis can be used for data reduction, simplifying complex data structures by identifying the most important underlying factors. It can also be used for hypothesis testing, to confirm or disconfirm theoretical models of relationships among variables.
There are two main types of factor analysis: exploratory factor analysis (EFA) and confirmatory factor analysis (CFA).
Exploratory factor analysis (EFA) is a statistical technique used to identify the underlying structure or dimensions that explain the correlations among a set of observed variables. The main goal of EFA is to reduce the complexity of a large set of variables by identifying a smaller number of factors that account for the observed variation.
Confirmatory factor analysis (CFA) is a statistical technique used to test a pre-specified factor structure or model of relationships among variables. Unlike exploratory factor analysis, which is used to identify the underlying structure of a set of variables, CFA is used to test a specific hypothesis or theory about the relationships among variables.
Assumptions in Factor Analysis
The following assumptions are made while using the factor analysis:
1. Data used in the factor analysis is based either on an interval or on a ratio scale.
2. Variables have a multivariate normal distribution.
3. The variables which have been selected in the study are relevant to the concept being assessed.
4. Enough sample size has been taken for factor analysis. Usually, a minimum of 10 observations per variable are required to run the factor analysis.
5. Outliers are not present in the data.
6. Some degree of collinearity exists among the variables but there should not be an extreme degree or singularity among the variables.
7. Linear relation exists among variables.
Characteristics
1. It provides the hidden dimensions of group characteristics that cannot be directly observed.
2. The procedure is straightforward and provides an opportunity to improve the model by using the explained variability of the group characteristics as a yardstick.
Factor
A factor is an underlying dimension that accounts for several observed variables. There can be one or more factors, depending upon the nature of the study and the number of variables involved in it.
Factor Loading
The correlation between the factor/component and independent variable is known as factor loading.
Factor loading is a statistical term that refers to the correlation between an observed variable and a latent factor in factor analysis. In factor analysis, the observed variables are thought to be influenced by one or more underlying factors that are not directly observable. Factor loading represents the strength of the relationship between each observed variable and the latent factor.
Factor loading values range from -1.0 to +1.0, with positive values indicating a positive relationship between the observed variable and the latent factor, and negative values indicating a negative relationship. The closer the factor loading value is to +1.0 or -1.0, the stronger the relationship between the observed variable and the latent factor. Factor loadings close to zero indicate that the observed variable is not strongly related to the latent factor.
Factor loadings are important in factor analysis because they provide information about the relative importance of each observed variable in measuring the underlying factor. Variables with high factor loadings on a particular factor are thought to be more closely related to that factor than variables with lower factor loadings. Factor loadings are also used to determine which observed variables should be included in a final factor solution, and to evaluate the reliability and validity of the factor solution.
Communality
Communality can be defined as the amount of variability in independent variables explained by all the identified factors in the model. In other words, communality represents the extent to which an observed variable shares a common variance with other variables in the factor analysis. Communality of any variable is obtained by adding the squared factor loadings of the variable on each factor and is represented by h^2.
h^2 of the ith variable = (ith factor loading of factor A)^2+ (ith factor loading of factor B^)2 + …
Communality values range from 0 to 1. Higher communality indicates the usefulness of the variable in explaining the group characteristics. On the other hand, low communality indicates that the identified factors in the model do not explain enough variability in the variable accordingly such variables should be removed from the analysis. Usually, the variable whose communality is less than 0.4 should be dropped. (1- communality = uniqueness)
Eigen value
The eigenvalue indicates the amount of variance of the independent variables explained by the factor. Eigen value is also referred to as characteristics root or latent root. The eigenvalue of a factor is obtained by summing the squares of all the factor loadings in that factor. Based on the magnitude of the eigenvalue, a decision about retaining the factor in the model is made. A higher eigenvalue magnitude indicates more usefulness of the factor in explaining the group characteristics. Eigen value indicates the relative importance of each factor in accounting for the particular set of variables being analyzed
Scree Plot
A scree plot is a graphical method used to determine the number of factors to retain in a factor analysis. It is named after the shape of the graph, which resembles the steep slope of a scree (rock debris) on a mountain.
To create a scree plot, you plot the eigenvalues of the factors in descending order on the y-axis, and the factor numbers on the x-axis. The resulting graph typically shows a steep drop in eigenvalues for the first few factors, followed by a more gradual decline. The point where the slope changes from steep to shallow is known as the "elbow point".
The scree plot can help to identify the number of factors to retain in factor analysis, as the elbow point represents the point where the added explanatory power of additional factors diminishes. Factors before the elbow point are considered significant, while those after the elbow point are considered to be of less importance.
Application of factor analysis in the theory and measurement of intelligence and personality
The concept of factor analysis was first introduced by the English mathematician and statistician Charles Spearman in 1904. He proposed that intelligence, as measured by IQ tests, is composed of two factors: "g" (general intelligence) and "s" (specific abilities). This idea laid the foundation for the development of factor analysis.
Factor analysis is commonly used in the measurement of intelligence to identify the underlying factors or dimensions that contribute to overall intellectual ability.
By administering a battery of intelligence tests to a large sample of individuals, researchers can use factor analysis to identify the common cognitive abilities or factors that are reflected in the test scores. These factors can then be used to develop a comprehensive measure of intelligence that incorporates a range of cognitive abilities.
Spearman's g-factor model of intelligence was developed using factor analysis, which he used to identify the underlying factors that contribute to overall intellectual ability. Spearman believed that intelligence was composed of both a general factor (g) and specific factors (s) that were related to particular cognitive abilities or tasks.
To develop his model, Spearman analyzed the results of a series of intelligence tests and found that there was a strong positive correlation between scores on different tests. He hypothesized that this correlation was due to the presence of a general factor of intelligence, or "g," that underlies performance on all cognitive tasks.
Spearman used factor analysis to identify this general factor by examining the patterns of correlations among the different tests. He found that a single factor, which he called "g," accounted for a large proportion of the variance in scores across different tests. This general factor was distinct from specific factors, which were related to performance on particular types of tasks.
Raymond Cattell's model of intelligence, known as the Cattell-Horn-Carroll (CHC) theory, also utilizes factor analysis to identify and describe different components or factors of intelligence.
Cattell used factor analysis to identify two primary factors of intelligence: fluid intelligence (Gf) and crystallized intelligence (Gc). Fluid intelligence is the ability to reason, think abstractly, and solve problems independent of prior knowledge or experience, while crystallized intelligence reflects the application of learned knowledge and skills.
Cattell then went on to further refine his model by using additional factor analysis to identify more specific factors of intelligence, such as visual-spatial ability, quantitative reasoning, and working memory. These specific factors were then integrated into the broader CHC model of intelligence, which incorporates both general and specific factors.
The use of factor analysis in this model has been instrumental in identifying the specific cognitive abilities that contribute to overall intellectual ability, as well as how these abilities are related to each other.
Louis L. Thurstone's model of intelligence is known as the multiple factor theory, which is based on the use of factor analysis to identify multiple distinct abilities that contribute to overall intellectual ability.
Thurstone used factor analysis to identify seven primary mental abilities: verbal comprehension, word fluency, number facility, spatial visualization, associative memory, perceptual speed, and reasoning. These abilities were identified based on the patterns of correlations among different tests of intelligence, with each ability representing a distinct factor or dimension of intelligence.
Thurstone's model of intelligence highlights the importance of specific abilities or factors, rather than a single general factor such as Spearman's g factor. This model suggests that each of the seven abilities identified by Thurstone contributes to intellectual ability and that individuals may excel in some abilities while struggling in others.
Factor analysis was instrumental in developing Thurstone's model of intelligence, as it allowed him to identify and isolate the distinct abilities that contribute to intellectual ability. The use of factor analysis in this model has been influential in highlighting the importance of specific abilities and dimensions of intelligence and has led to further research on the nature of intelligence and the cognitive processes that contribute to it.
J.P. Guilford's theory of intelligence, known as the Structure of Intellect model, also used factor analysis to identify and describe the underlying factors or dimensions of intelligence.
Guilford's model posits that there are three primary dimensions of intellectual functioning: operations, contents, and products. Operations refer to the mental processes or cognitive abilities used to perform tasks, contents refer to the specific information or knowledge that is processed by these operations, and products refer to the outcomes or results of the processing.
Within each of these three dimensions, Guilford identified multiple sub-factors using factor analysis. For example, under operations, Guilford identified 5 types of mental operations: cognition, memory, divergent thinking, convergent thinking, and evaluation. Within these sub-factors, Guilford identified multiple specific abilities or components that contributed to the overall factor.
Factor analysis played a critical role in Guilford's model, as it allowed him to identify and isolate the specific cognitive abilities or components that contribute to each factor. By using factor analysis to analyze the correlations among different measures of intelligence, Guilford was able to identify the specific abilities that were most strongly associated with each factor.
Overall, Guilford's Structure of Intellect model highlights the importance of specific cognitive abilities and components in understanding intellectual functioning, and factor analysis plays a crucial role in identifying these components and organizing them into a comprehensive framework.
John Carroll's three-stratum theory is another model of intelligence that relies heavily on factor analysis to identify the underlying structure of intellectual abilities.
Carroll's theory posits that there are three levels, or strata, of cognitive abilities:
Stratum I includes narrow, specific abilities that are highly specialized and typically measured by specific tests. These abilities include things like spatial perception, verbal comprehension, and numerical ability.
Stratum II includes broad abilities that are composed of multiple specific abilities. These include factors like fluid intelligence, crystallized intelligence, and visual processing.
Stratum III includes general intelligence, or g, which is the highest level of intellectual functioning and is composed of combinations of the broad abilities at Stratum II.
Factor analysis played a key role in Carroll's three-stratum theory, as it was used to identify and describe the specific abilities at each level of analysis. By analyzing the patterns of correlations among different measures of intellectual abilities, Carroll was able to identify the specific factors or dimensions that contribute to each ability.
For example, factor analysis was used to identify the specific cognitive abilities that contribute to fluid intelligence, such as working memory, perceptual speed, and spatial visualization. These abilities were then combined into a broad factor at Stratum II, and the correlations among these broad factors were used to identify the general factor of intelligence at Stratum III.
Overall, Carroll's three-stratum theory represents a comprehensive and well-organized model of intelligence that relies heavily on factor analysis to understand the underlying structure of cognitive abilities.
Personality
Factor analysis is also commonly used in theories of personality to identify and describe the underlying dimensions of personality traits. To develop a personality inventory, researchers typically begin by generating a large pool of potential items that are thought to be related to different aspects of personality. These items are then administered to a large sample of participants, and their responses are analyzed using factor analysis.
Factor analysis is used to identify the patterns of correlations among the different items. Highly correlated items are considered to reflect the same underlying dimension of personality, and these items are combined into a scale or subscale that represents that dimension.
For example, if items related to being outgoing, sociable, and assertive are highly correlated, they may be combined into a scale that represents the dimension of extraversion. Similarly, if items related to being organized, reliable, and diligent are highly correlated, they may be combined into a scale that represents the dimension of conscientiousness.
Once the underlying dimensions of personality have been identified, researchers can use the inventory to assess the levels of each dimension in individuals or groups. This can be useful for a variety of purposes, such as understanding individual differences in personality, predicting behavior or outcomes, or identifying areas for personal growth or development.
The Five-Factor Model (FFM) of personality, also known as the Big Five personality traits, was developed using factor analysis. Factor analysis was used to identify the underlying dimensions of personality that are most important in describing individual differences. The five factors, which are the basis of the FFM, are:
- Openness to Experience: This factor captures an individual's willingness to engage with new experiences and ideas, as well as their intellectual curiosity, creativity, and imagination.
- Conscientiousness: This factor reflects an individual's level of organization, self-discipline, and reliability, as well as their ability to plan and achieve long-term goals.
- Extraversion: This factor captures an individual's level of sociability, assertiveness, and emotional expressiveness, as well as their tendency to seek out stimulation and excitement.
- Agreeableness: This factor reflects an individual's level of kindness, empathy, and cooperativeness, as well as their tendency to avoid conflict and seek social harmony.
- Neuroticism: This factor reflects an individual's tendency toward negative emotions such as anxiety, depression, and anger, as well as their level of emotional instability and sensitivity.
Factor analysis has been used extensively in the development and validation of the FFM. Researchers have used factor analysis to identify the most important personality traits, as well as to refine and validate measures of those traits. Factor analysis has also been used to explore the structure of personality across different cultures and demographic groups, and to investigate the relationships between personality traits and a wide range of outcomes, such as job performance, academic achievement, and mental health.
Hans Eysenck's Three Factor Model of personality is another widely studied and accepted model of personality that uses factor analysis. Eysenck's model posits that three primary factors underlie human personality, which he referred to as:
- Extraversion: This factor is characterized by outgoingness, assertiveness, and sociability.
- Neuroticism: This factor is characterized by emotional instability, anxiety, and moodiness.
- Psychoticism: This factor is characterized by traits such as impulsivity, aggression, and a lack of empathy.
Factor analysis was used to identify these three factors as the most important underlying dimensions of personality in Eysenck's model. Eysenck believed that these three factors were largely biologically determined and that they had significant implications for a wide range of outcomes, including health, behavior, and social functioning. Factor analysis was also used to develop and validate measures of each of these three factors. Eysenck and his colleagues developed a widely used personality questionnaire, known as the Eysenck Personality Questionnaire (EPQ), which uses factor analysis to measure an individual's level of extraversion, neuroticism, and psychoticism.
Since its inception, Eysenck's Three Factor Model has been widely studied and validated in numerous cultures and demographic groups. While some researchers have raised concerns about the validity and generalizability of the model, it remains an important and influential approach to understanding human personality.
Raymond Cattell's 16 Personality Factor (16PF) model is another example of a personality theory that uses factor analysis. Cattell's model posits that 16 underlying dimensions of personality can be used to describe individual differences. These 16 factors are:
Warmth, Reasoning, Emotional Stability, Dominance, Liveliness, Rule-Consciousness, Social Boldness, Sensitivity, Vigilance, Abstractedness, Privateness, Apprehension, Openness to Change, Self-Reliance, Perfectionism, Tension
Factor analysis was used to identify and validate these 16 factors as the most important underlying dimensions of personality in Cattell's model. The initial version of the 16PF questionnaire was developed using a combination of factor analysis and theoretical reasoning, and subsequent versions of the questionnaire were refined and validated using factor analysis.
Factor analysis has also been used to investigate the relationships between the 16PF factors and other important outcomes, such as job performance, academic achievement, and mental health. For example, research has found that individuals who score high on the Emotional Stability factor tend to have better mental health outcomes, while individuals who score high on the Dominance factor tend to be more successful in leadership roles.
While the 16PF model has been widely used and validated, some researchers have raised concerns about its complexity and its ability to capture the full range of human personality. Nonetheless, the model remains an important and influential approach to understanding individual personality differences.
Limitations of factor analysis
1. The analysis provides good results only if all the relevant variables that measure the group characteristics are included in the study.
2. In a situation where the majority of variables are highly related, the factor analysis may club them into one factor. This will not allow other factors to be identified in the model that might capture more useful relationships.
3. Using factor analysis in constructing psychological tests requires a good domain knowledge for identifying and naming factors because many times multiple variables can be highly related without any reason.

Hey there, curious minds! I'm Sayani Banerjee, and I'm thrilled to be your companion on the fascinating journey through the realm of psychology. As a dedicated student pursuing my master's in Clinical Psychology at Calcutta University, I'm constantly driven by the desire to unravel the mysteries of the human mind and share my insights with you. My passion for teaching and my love for research come together on my blog, psychologymadeeasy.in, where we explore the world of psychology in the simplest and most engaging way possible.